Resumes
Resumes
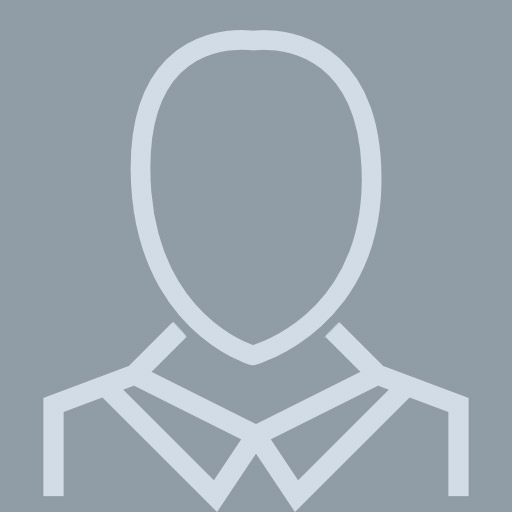
Associate Professor
View pageLocation:
140 Homestead Cir, Ithaca, NY 14850
Industry:
Higher Education
Work:
Washington University in St. Louis - Saint Louis since Jan 2010
Assistant Professor
Yahoo! Jul 2007 - Jan 2010
Research Scientist
IBM May 2006 - Aug 2007
Research Internship
Assistant Professor
Yahoo! Jul 2007 - Jan 2010
Research Scientist
IBM May 2006 - Aug 2007
Research Internship
Education:
University of Pennsylvania 2003 - 2007
PhD, Machine Learning University of Pennsylvania 2003 - 2004
MSc, Computer Science University of Oxford 1999 - 2002
PhD, Machine Learning University of Pennsylvania 2003 - 2004
MSc, Computer Science University of Oxford 1999 - 2002
Skills:
Machine Learning
Algorithms
Artificial Intelligence
Pattern Recognition
Data Mining
Natural Language Processing
Algorithms
Artificial Intelligence
Pattern Recognition
Data Mining
Natural Language Processing