Resumes
Resumes
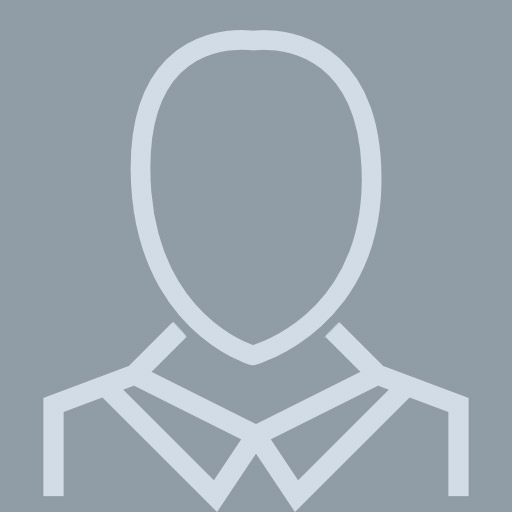
Siddharth Khullar Redmond, WA
View pageWork:
Quanttus, Inc.
Mar 2014 to 2000
Principal Data Scientist
Microsoft Research
Jan 2013 to 2000
Post-Doctoral Researcher
Microsoft
Jun 2012 to 2000
Research Intern (MSR)
Mind Research Network for Neurodiagnostic Discovery
Jun 2009 to 2000
Graduate Research Associate, Medical Image Analysis Group
MIT Media Lab, Massachusetts Institute of Technology
Cambridge, MA
Sep 2011 to Jun 2012
Research Assistant, Camera Culture Group
Digital Imaging and Printing Labs, RIT, NY, USA
Mar 2008 to May 2009
Research Assistant
Signal Processing and WiComm Labs at NIEC
Delhi, Delhi
Jan 2006 to Jun 2007
Research Assistant, Multimedia Application Development
Mar 2014 to 2000
Principal Data Scientist
Microsoft Research
Jan 2013 to 2000
Post-Doctoral Researcher
Microsoft
Jun 2012 to 2000
Research Intern (MSR)
Mind Research Network for Neurodiagnostic Discovery
Jun 2009 to 2000
Graduate Research Associate, Medical Image Analysis Group
MIT Media Lab, Massachusetts Institute of Technology
Cambridge, MA
Sep 2011 to Jun 2012
Research Assistant, Camera Culture Group
Digital Imaging and Printing Labs, RIT, NY, USA
Mar 2008 to May 2009
Research Assistant
Signal Processing and WiComm Labs at NIEC
Delhi, Delhi
Jan 2006 to Jun 2007
Research Assistant, Multimedia Application Development
Education:
Chester F. Carlson Center for Imaging Science
Rochester, NY
2009 to 2013
PhD in Imaging Science
Rochester Institute of Technology
Rochester, NY
2007 to 2009
M.S. in Electrical Engineering
G.G.S Indraprastha University
New Delhi, Delhi
2003 to 2007
Bachelor of Technology in Electrical Engineering
Rochester, NY
2009 to 2013
PhD in Imaging Science
Rochester Institute of Technology
Rochester, NY
2007 to 2009
M.S. in Electrical Engineering
G.G.S Indraprastha University
New Delhi, Delhi
2003 to 2007
Bachelor of Technology in Electrical Engineering
Skills:
Programming languages: MATLAB, MEX interfacing, C/C++ (OpenCV), Beginner in Android SDK, iOS SDK. Design/Scripting Tools: Adobe Creative Suite3+, MS Visio, Office, LaTeX, Python. Neuroimaging Tools: SPM, AFNI, Group-ICA Toolbox (GIFT), MRICro. OS: Linux (Ubuntu, CentOS), Windows, OS-X.