Resumes
Resumes
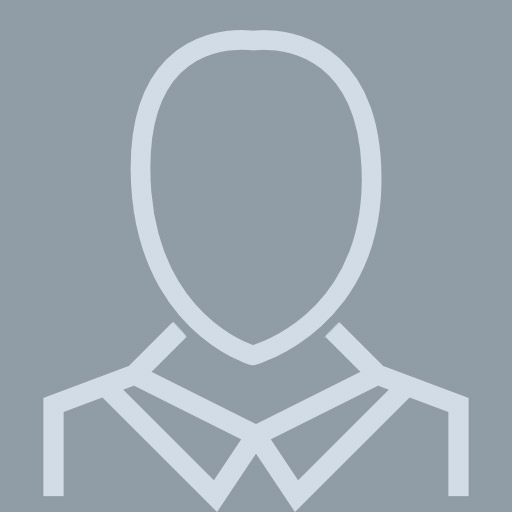
Buffalo Grove High School
View pageLocation:
451 Shoreline Blvd north, Mountain View, CA 94041
Industry:
Legal Services
Work:
Casetext May 2019 - Jan 2020
Product Manager
P.i.n.g. Inc. Feb 2017 - May 2017
Data Science Intern
Hp May 2016 - May 2017
Product Management Intern
Legalforce Rapc Jan 2013 - Jan 2016
Senior Trademark Prosecution Specialist
Legalforce Rapc Feb 2013 - Aug 2015
Business Development
Product Manager
P.i.n.g. Inc. Feb 2017 - May 2017
Data Science Intern
Hp May 2016 - May 2017
Product Management Intern
Legalforce Rapc Jan 2013 - Jan 2016
Senior Trademark Prosecution Specialist
Legalforce Rapc Feb 2013 - Aug 2015
Business Development
Education:
University of California, Berkeley 2015 - 2017
Bachelors, Bachelor of Arts, Economics, Computer Science, Applied Mathematics De Anza College 2013 - 2015
Buffalo Grove High School
Bachelors, Bachelor of Arts, Economics, Computer Science, Applied Mathematics De Anza College 2013 - 2015
Buffalo Grove High School
Skills:
Microsoft Office
Microsoft Excel
Microsoft Word
Customer Service
Powerpoint
English
Research
Windows
Teaching
Public Speaking
Budgets
Strategic Planning
Trademarks
Trademark Infringement
Copyright Law
Intellectual Property
Microsoft Excel
Microsoft Word
Customer Service
Powerpoint
English
Research
Windows
Teaching
Public Speaking
Budgets
Strategic Planning
Trademarks
Trademark Infringement
Copyright Law
Intellectual Property
Languages:
English
Gujarati
Hindi
Gujarati
Hindi
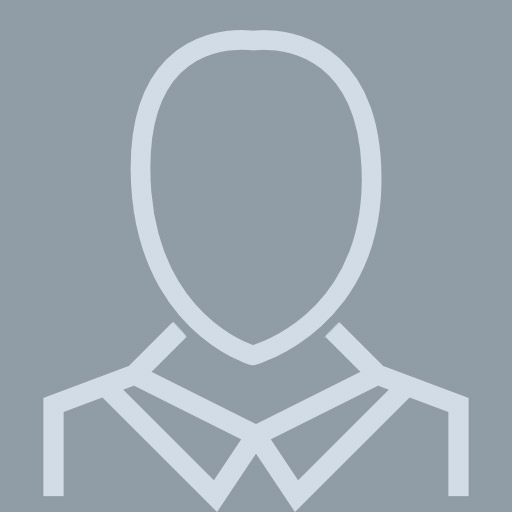
Ravi Soni
View page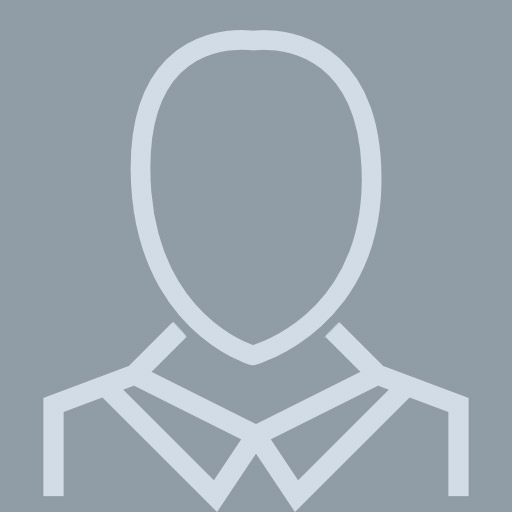
Ravi Soni
View page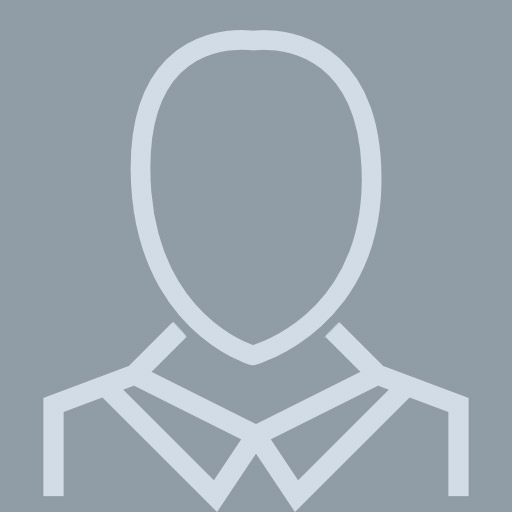
Ravi Soni
View pageLocation:
United States
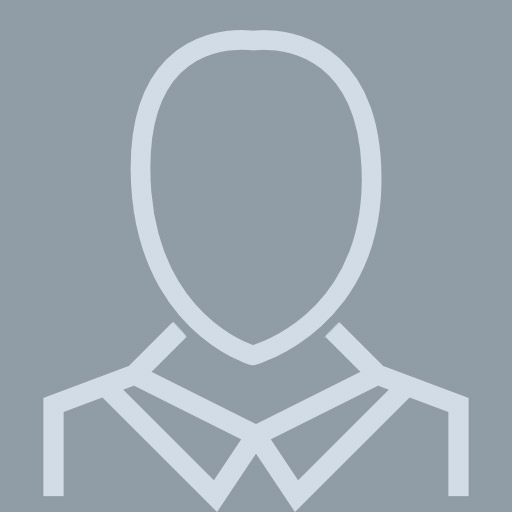
Ravi Soni
View pageLocation:
United States