Resumes
Resumes
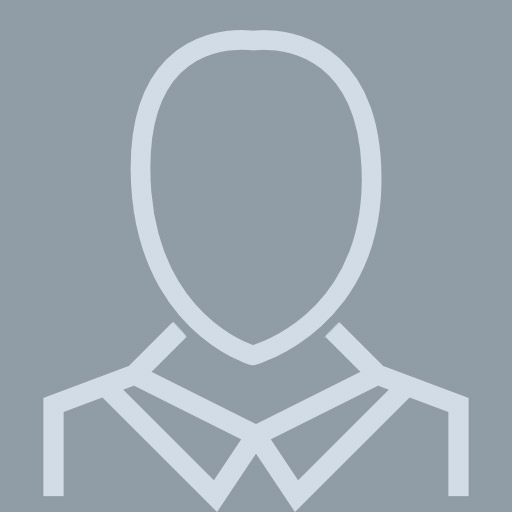
Senior Machine Learning Researcher
View pageLocation:
San Francisco, CA
Industry:
Information Technology And Services
Work:
Twitter
Senior Machine Learning Researcher
Twitter Jun 2017 - Jan 2019
Senior Machine Learning Engineer
Silicon Villas Jun 2017 - Jan 2019
Python Instructor
Conversant Llc Sep 2015 - Jun 2017
Senior Scientist
University of Rome "Tor Vergata" Dec 2016 - Dec 2016
Seminar: A Gentle Introduction To Machine Learning
Senior Machine Learning Researcher
Twitter Jun 2017 - Jan 2019
Senior Machine Learning Engineer
Silicon Villas Jun 2017 - Jan 2019
Python Instructor
Conversant Llc Sep 2015 - Jun 2017
Senior Scientist
University of Rome "Tor Vergata" Dec 2016 - Dec 2016
Seminar: A Gentle Introduction To Machine Learning
Education:
University of Rome Tor Vergata 2008 - 2013
Doctorates, Doctor of Philosophy, Mathematics, Philosophy Instituto Nacional De Matemática Pura E Aplicada 2010 - 2011
Doctorates, Doctor of Philosophy, Mathematics Master In Data Intelligence E Strategie Decisionali - La Sapienza 2006 - 2008
Master of Science, Masters, Mathematics Heidelberg University 2006 - 2007
Master In Data Intelligence E Strategie Decisionali - La Sapienza 2003 - 2006
Bachelors, Bachelor of Science, Mathematics Università Di Roma Tor Vergata
Doctorates, Doctor of Philosophy, Mathematics, Philosophy Instituto Nacional De Matemática Pura E Aplicada 2010 - 2011
Doctorates, Doctor of Philosophy, Mathematics Master In Data Intelligence E Strategie Decisionali - La Sapienza 2006 - 2008
Master of Science, Masters, Mathematics Heidelberg University 2006 - 2007
Master In Data Intelligence E Strategie Decisionali - La Sapienza 2003 - 2006
Bachelors, Bachelor of Science, Mathematics Università Di Roma Tor Vergata
Skills:
Mathematica
Mathematical Modeling
Programming
Mathematics
Statistics
Machine Learning
Latex
Python
Mathematics Education
C++
Physics
Wolfram Language
Data Analysis
Problem Solving
R
Scientific Computing
Amazon Web Services
Deep Learning
Natural Language Processing
Computer Vision
Cvs
Git
Scala
Mathematical Modeling
Programming
Mathematics
Statistics
Machine Learning
Latex
Python
Mathematics Education
C++
Physics
Wolfram Language
Data Analysis
Problem Solving
R
Scientific Computing
Amazon Web Services
Deep Learning
Natural Language Processing
Computer Vision
Cvs
Git
Scala
Languages:
Italian
English
German
Portuguese
French
English
German
Portuguese
French
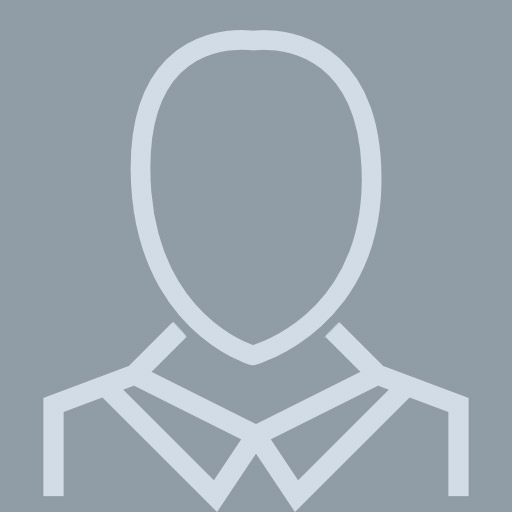
Luca Belli
View page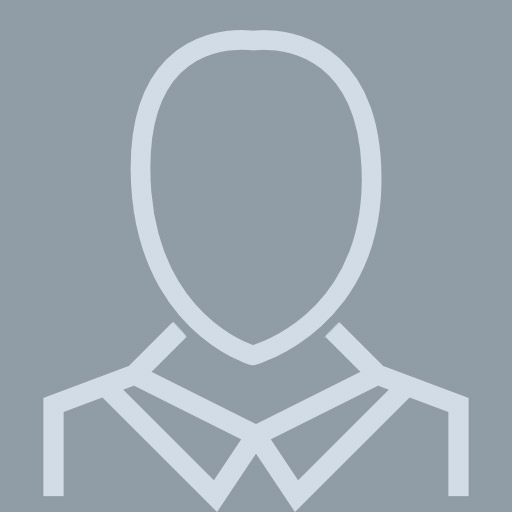