Resumes
Resumes
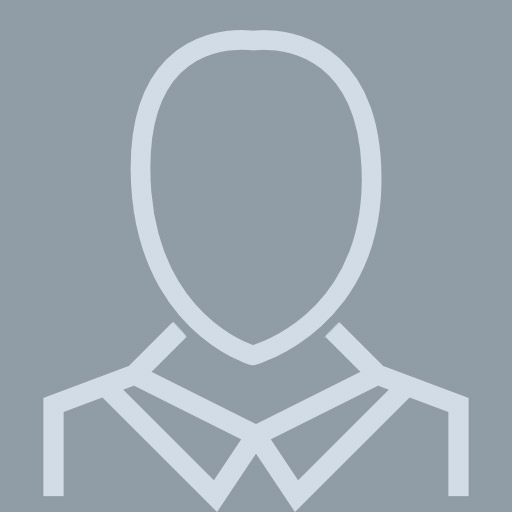
Senior Scientist At Siemens
View pageLocation:
37b Melrose Ct, Princeton, NJ 08540
Industry:
Computer Software
Work:
Siemens
Senior Scientist at Siemens
Siemens Oct 2010 - Dec 2014
Research Scientist
Sas Oct 2004 - Sep 2010
Operations Research Specialist
Princeton University Sep 2001 - Sep 2004
Postdoctoral Research Associate
Imperial College London Sep 1999 - Aug 2001
Research Assistant
Senior Scientist at Siemens
Siemens Oct 2010 - Dec 2014
Research Scientist
Sas Oct 2004 - Sep 2010
Operations Research Specialist
Princeton University Sep 2001 - Sep 2004
Postdoctoral Research Associate
Imperial College London Sep 1999 - Aug 2001
Research Assistant
Education:
Dyson School of Design Engineering 1995 - 2000
Doctorates, Doctor of Philosophy, Computer Science The University of Manchester 1991 - 1992
Master of Science, Masters, Computer Science Aristotle University of Thessaloniki (Auth) 1986 - 1990
Bachelors, Bachelor of Science, Mathematics
Doctorates, Doctor of Philosophy, Computer Science The University of Manchester 1991 - 1992
Master of Science, Masters, Computer Science Aristotle University of Thessaloniki (Auth) 1986 - 1990
Bachelors, Bachelor of Science, Mathematics
Skills:
Algorithms
Mathematical Modeling
Operations Research
Data Mining
Machine Learning
Statistics
Data Analysis
Optimization
C++
Statistical Modeling
High Performance Computing
Sas
Artificial Intelligence
R
Latex
Mathematical Modeling
Operations Research
Data Mining
Machine Learning
Statistics
Data Analysis
Optimization
C++
Statistical Modeling
High Performance Computing
Sas
Artificial Intelligence
R
Latex