Resumes
Resumes
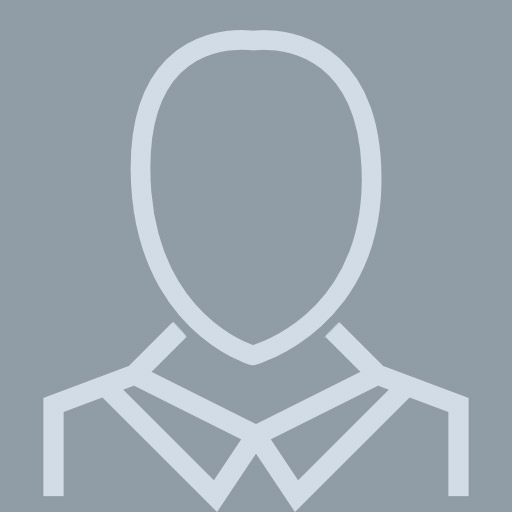
Hui Zhou Las Vegas, NV
View pageWork:
Casino M8trix
San Jose, CA
2012 to May 2014
table games dealer
California Grand Casino
Pleasant Hill, CA
2005 to Aug 2008
Poker Dealer
SourceCorp
2002 to Sep 2004
Data Entry
San Jose, CA
2012 to May 2014
table games dealer
California Grand Casino
Pleasant Hill, CA
2005 to Aug 2008
Poker Dealer
SourceCorp
2002 to Sep 2004
Data Entry
Education:
Let's Make A Dealer Poker Training School
Las Vegas, NV
2015 to 2015
Certificate
City College of San Francisco
2001 to 2002
Certificate in Accounting and office clerk
YunNan University
1978 to 1981
Physics Graduate for AS
Las Vegas, NV
2015 to 2015
Certificate
City College of San Francisco
2001 to 2002
Certificate in Accounting and office clerk
YunNan University
1978 to 1981
Physics Graduate for AS
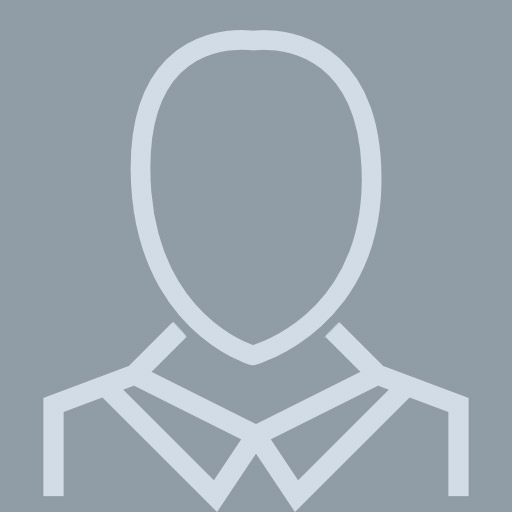
Hui Zhou San Ramon, CA
View pageWork:
Theranos
Palo Alto, CA
Mar 2011 to Nov 2011
Sr. Scientist
Bio-Rad Laboratories
Hercules, CA
2005 to 2011
Scientist
Zyomyx, Inc
Hayward, CA
2001 to 2004
Associate Scientist
Qiagen-Operon Technologies, Inc
Alameda, CA
2000 to 2001
Scientist
AlphaGene Inc
Woburn, MA
1998 to 2000
Senior Research Associate
Genome Therapeutics Inc
Waltham, MA
1997 to 1998
Research Associate
Palo Alto, CA
Mar 2011 to Nov 2011
Sr. Scientist
Bio-Rad Laboratories
Hercules, CA
2005 to 2011
Scientist
Zyomyx, Inc
Hayward, CA
2001 to 2004
Associate Scientist
Qiagen-Operon Technologies, Inc
Alameda, CA
2000 to 2001
Scientist
AlphaGene Inc
Woburn, MA
1998 to 2000
Senior Research Associate
Genome Therapeutics Inc
Waltham, MA
1997 to 1998
Research Associate
Education:
The University of Mississippi, University, MS
1993
Master of Science in Biochemistry
Fudan University
Jan 1987
Bachelor of Science in Biochemistry
1993
Master of Science in Biochemistry
Fudan University
Jan 1987
Bachelor of Science in Biochemistry
Skills:
process/assay development, molecular biology, microarray
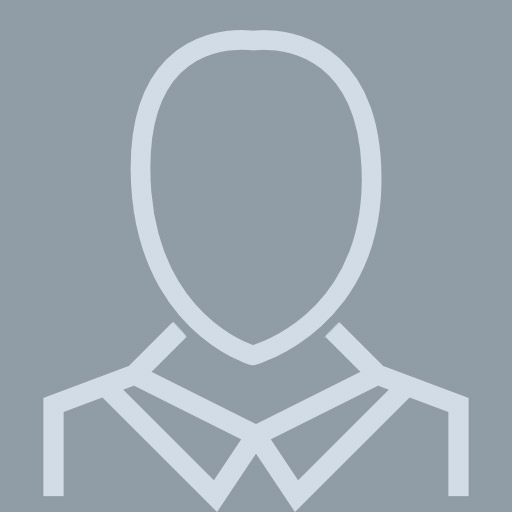
Hui Zhou Belmont, CA
View pageWork:
Vanderbilt University
2011 to 2000
Bioinformatics Manager
University of California at Los Angeles
Los Angeles, CA
2006 to 2011
Senior Statistician
University of Texas Southwestern Medical Center
Dallas, TX
2005 to 2006
Senior Research Associate
Molecular Kinetics Inc
Indianapolis, IN
2003 to 2005
Computational Biologist
Celltech R&D Inc., Bioinformatics Group
Seattle, WA
2002 to 2002
Intern
Children's Hospital of Los Angeles
Los Angeles, CA
2001 to 2002
Research Assistant
University of California
San Francisco, CA
2001 to 2001
Research Intern
University of Missouri
Kansas City, MO
1999 to 2000
Research Assistant
Chinese Academy of Medical Sciences, National Heart Institute
1995 to 1999
Resident Cardiologist
2011 to 2000
Bioinformatics Manager
University of California at Los Angeles
Los Angeles, CA
2006 to 2011
Senior Statistician
University of Texas Southwestern Medical Center
Dallas, TX
2005 to 2006
Senior Research Associate
Molecular Kinetics Inc
Indianapolis, IN
2003 to 2005
Computational Biologist
Celltech R&D Inc., Bioinformatics Group
Seattle, WA
2002 to 2002
Intern
Children's Hospital of Los Angeles
Los Angeles, CA
2001 to 2002
Research Assistant
University of California
San Francisco, CA
2001 to 2001
Research Intern
University of Missouri
Kansas City, MO
1999 to 2000
Research Assistant
Chinese Academy of Medical Sciences, National Heart Institute
1995 to 1999
Resident Cardiologist
Education:
Shandong University School of Medicine
Jinan, Shandong Province, P. R. China
1990 to 1995
MD in Medicine
University of Southern California
Los Angeles, CA
M. S. in Computational Biology and Bioinformatics
Jinan, Shandong Province, P. R. China
1990 to 1995
MD in Medicine
University of Southern California
Los Angeles, CA
M. S. in Computational Biology and Bioinformatics
Skills:
COMPUTER SKILLS Experienced in the application of: Database and Software: Database (MySQL, SQL server, ORACLE), data analysis (R/Bioconductor, Matlab, SAS), bioinformatics (BLAST, CASAVA, BWA, GATK, SAMtools, Picard, Bowtie, TopHat, Cufflinks, GeneSpring, Partek, Ingenuity, DAVID, Galaxy, GenePattern, CLUSTALW, PSI-Pred, MASCOT, Phrap, MEME suite, Cytoscape) as well as online tools and databases (NCBI, UCSC genome browser) OS and Programming: LINUX, Windows, PERL/DBI, CGI, C++, Python, shell, JAVA Script, Coldfusion Ontology, data exchange standards and semantic web technologies: GO, XML. High performance computing: TORQUE PBS