Resumes
Resumes
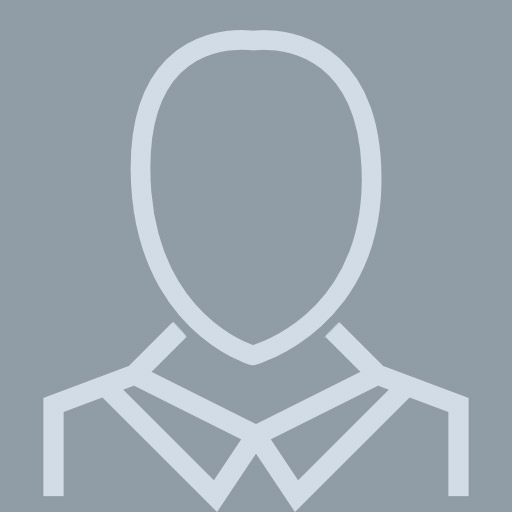
Member Of Technical Staff
View pageLocation:
Austin, TX
Industry:
Computer Hardware
Work:
Amd
Member of Technical Staff
Qualcomm
Senior Engineer
Arm Jul 2015 - Oct 2016
Senior Research Engineer
Qualcomm May 2013 - Aug 2013
Cpu R and D Intern
Ibm May 2012 - Apr 2013
Performance and Energy Modeling Intern
Member of Technical Staff
Qualcomm
Senior Engineer
Arm Jul 2015 - Oct 2016
Senior Research Engineer
Qualcomm May 2013 - Aug 2013
Cpu R and D Intern
Ibm May 2012 - Apr 2013
Performance and Energy Modeling Intern
Education:
University of Wisconsin - Madison 2009 - 2015
Doctorates, Masters, Doctor of Philosophy, Computer Engineering, Philosophy Bucknell University 2005 - 2009
Bachelors, Electrical Engineering
Doctorates, Masters, Doctor of Philosophy, Computer Engineering, Philosophy Bucknell University 2005 - 2009
Bachelors, Electrical Engineering
Skills:
Computer Architecture
Verilog
Processors
Matlab
Simulations
Fpga
Xilinx
Vlsi
Functional Verification
C++
Embedded Systems
Perl
Research
Latex
Verilog
Processors
Matlab
Simulations
Fpga
Xilinx
Vlsi
Functional Verification
C++
Embedded Systems
Perl
Research
Latex
Languages:
English
French
French